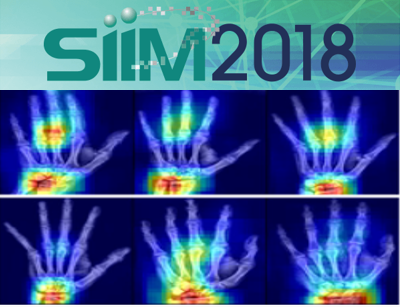
What Machines Can Read: Gender Identification from Hand and Wrist Radiographs in Children (SIIM 2018 Presentation)
Sehyo Yune (MD, MPH, MBA) gave a presentation on her paper “What Machines Can Read: Gender Identification from Hand and Wrist Radiographs in Children” at SIIM 2018 Annual Meeting.
Yune, S., Lee, H., Kim, M., Tajmir, S., Gee, M., Lev, M., Do, S., 2018. What Machines Can Read: Gender Identification from Hand and Wrist Radiographs in Children. In Proceedings of the Society for Imaging Informatics in Medicine Annual Meeting.
Machine learning extracts meaningful patterns from medical images without use of explicit hand-crafted features. This approach shed light on finding patterns that have been previously beyond human visual perception to detect an early stage disease or discover new insights. Skeletal sexual dimorphism develops mostly in pelvis during puberty. In hand and wrist, prior work has shown higher second-to-fourth digit ratio (2D:4D) and smaller carpal bones in females compared to males. However, no discrete feature has been described in hand and wrist that distinguishes male from female, either in children or adults. To explore the ability of deep learning algorithms to detect subtle differences in medical images that are not recognized by human radiologists, we trained and tested a deep convolutional neural network (CNN) with hand and wrist radiographs of children to predict gender.