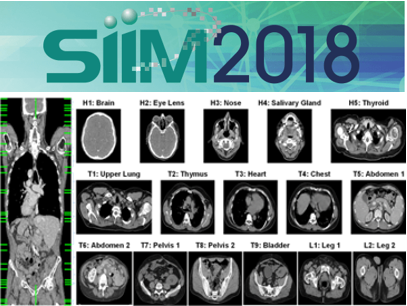
Machine Friendly Machine Learning: Deep Dive into Raw Data Domain for Medical Image Analysis (SIIM 2018 Presentation)
Hyunkwang Lee (Ph.D. Candidate in Electrical Engineering, Harvard School of Engineering and Applied Sciences) gave a presentation on his paper “Machine Friendly Machine Learning: Deep Dive into Raw Data Domain for Medical Image Analysis” at SIIM 2018 Annual Meeting.
Lee, H., Yune, S., Kim, M., Brady, T., Lev, M., Do, S., 2018. Machine Friendly Machine Learning: Deep Dive into Raw Data Domain forMedical Image Analysis. In Proceedings of the Society for Imaging Informatics in Medicine Annual Meeting.
The recent advancement in deep learning to recognize meaningful features from various forms of data opened the door to numerous applications in artificial intelligence (AI) for medical image analysis. However, to date, only the images carefully reconstructed from signals generated by image acquisition system can be utilized to build AI systems. In this study, we developed a deep learning system for human anatomy identification and intracranial hemorrhage (ICH) detection using sinograms, a mathematical representation of projected ray-sums of Radon space, and compared its performance to the system based on reconstructed computed tomography (CT) images.